Article begins
In August 2023, during the first Republican primary debate, nominee Chris Christie unleashed an insult directed at rival Vivek Ramaswamy: “Hold on, I’ve had enough already tonight of a guy who sounds like ChatGPT!” The crowd laughed and jeered. In the space of just nine months, ChatGPT had, apparently, developed a “voice” that was recognizable by a sizeable chunk of the US population. OpenAI’s ChatGPT had been reduced to a punchline—a trope revealing robotic, repetitive, and over-confident talk.
For anthropologists interested in both language and science studies, this period of rapid growth in the subfield of AI research known as natural language processing (NLP) has been fascinating. Discoveries that were usually of interest to a handful of computer scientists are now spawning products and memes that circulate widely. “People feel like they interacted with an algorithm for the first time,” says one of my contacts, a machine learning scientist from Silicon Valley, about the astonishment people feel when interacting with ChatGPT. On social media, at dinner parties, and in the halls of academia, suddenly everyone has a theory of language and what role it plays in structuring human, and maybe computer, thought. People’s language ideologies are being tested, questioned, and often rejigged by these interactions. The public is in the process of, to use Lucy Suchman’s term, “refiguring” the dividing line between humans and machines.
My own research looks at how AI scientists make sense of the work they are doing and how they communicate complex and abstract concepts across fields of study. Scientists working in the field of NLP are also recalibrating the basic assumptions of their field through a process that involves arguments laid out in journal articles and heated debates at conferences. At a machine learning conference I attended in June 2023, the opening speaker described his interactions with the newly released GPT4 as “a religious experience.” “Nobody was expecting this,” said someone at my table when I asked him about the success of these recent large language models (LLMs). “How are they doing so well with decoder-only models?” he continued, mostly to himself, referring to the type of algorithm that compose these generative AI tools. The speaker put up another slide with a tweet from a famous investor in Silicon Valley: “Guys. Existential crisis. Did OpenAI just finish software? What’s there left to do but clean up and sweep?” One computational linguist I know had a grant application denied because, in the words of a grant reviewer, “language has been solved.” The scientists like this that work on AI tools are often rendered invisible by tech company marketing. But, as science and technology studies (STS) scholars know well, there are people hidden all the way down the “tech stack.” People write the code comprising the models, they write the text upon which LLMs are trained, they “fine-tune” the models by providing explicit feedback on what it got right or wrong, and they filter out inappropriate content from both training sets and user-inputted data. As Nick Seaver says, “algorithms are human, too.”
The invisible humans who make LLMs possible stand in stark contrast to the near-mythical figure of the elder statesmen of artificial intelligence, such as neural net scientist Geoff Hinton, usually referred to in the media with the honorific “the godfather of AI.” On the conference circuit, scientists were eager to talk about Hinton’s decision to quit his lucrative position at Google (in May 2023) to speak out about the “existential threat” he saw in generative AI. As a prophet bringing warning, Hinton spoke in person at some of these conferences, explaining that AI was becoming “superintelligent” and could conceivably wrest control of society from humans. He often structured his talks as rebuttals to his most strident critics. His foils included philosophers, linguists, and other AI researchers (some of them good friends of his) who disagreed with his assessment of AI risk. These conferences provided a platform for Hinton, as well as thousands of other presenters in much smaller conference rooms, to show their commitment to the dialogic engine of scientific progress. Another way of thinking about these disagreements is as an example of what Victor Turner calls a “social drama,” one that originated in the arcane halls of academia but was now rippling out into the popular media.
Social dramas play out as “a trial of strength between influential paradigm-bearers,” writes Turner. Bruno Latour frames scientists as “spokesmen” for theories (and, especially in AI, they are almost always men), defending their version of the facts in trials of strengths. “Some of these trials are imposed . . . by the scientific objector” or “dissenter,” he writes, a moniker one imagines Hinton would wear with pride. The formal debate, the academic lecture, the submission of an LLM into a benchmarking competition are all “trials of strength” for both the models of NLP as well as for their proxies, the scientists themselves.
Many of these trials of strength run in circles. Adversaries often argue past each other, demonstrating the idea from ordinary language philosophy that most philosophical disagreements stem from differences in how people use the same words. This gap in meaning applies, in an appropriately self-referential manner, to the concept of language itself. In computational linguistics, language is treated as a symbolic system of words with fixed meanings that map neatly onto the world. For anthropologists, though, social context is a prerequisite for meaning to emerge from words. Speakers rely not so much on symbols with fixed meanings but on “indexes” that represent social types or norms. The papers at the computational linguistics conference I attended treated paralinguistic features like intonation, body language, gaze, and tone of voice as distractions. The ambiguity of pronouns like him or there, which depend on social context for their meaning, are treated as problems to be solved through the application of more rigorous logic or, the new rallying cry, “more data!” Often, these features of language were, with echoes of Mary Douglas, described as “messy.” The data sets needed to be “cleaned up” before being pressed into service to train a model.
Over the past few months, to get a sense of what it’s like to “clean up” messy data, I have been working with not-for-profit Cohere For AI toward the goal of releasing an open-source, multilingual data set and a fully trained language model. The Aya project is committed to including a diverse set of languages in their data; their unofficial motto is “no language left behind.” The internet, as anyone who lives outside of North America is painfully aware, is mostly composed of English content—64 percent of it to be exact, while only 5 percent of the world’s population speak English at home. This alienates a huge chunk of the world’s population from the tools that spin out of NLP. “Technology is going to make it way easier for people to adopt some languages more than others,” says Sara Hooker, who left a job at Google Research to become the head of Cohere For AI last year, “but the current models only really serve English.” The Aya data set has over 100 languages. It’s a small percentage of the world’s languages, yet when complete, it will be the most diverse, multilingual, open-source data set released to date. “This is urgent, and we need to make technology that serves other languages,” says Hooker, “it’s a band-aid solution, but we’ve got to start somewhere.”
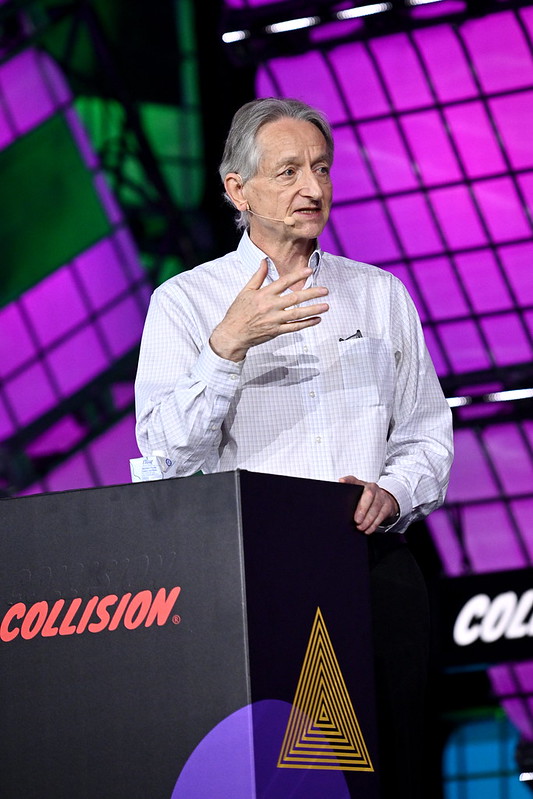
My role, with a team of volunteers, is to help “fine-tune” the language model, a process that relies on human feedback to nudge the model into creating better responses. I write prompts and responses, the kind you might expect from ChatGPT, in French. From these examples, the model “learns” how to write “good” answers. There are teams doing this in other languages, too. Nathanaël Carraz Rakotonirina heads up the Malagasy team from his apartment in Barcelona at the Universitat Pompeu Fabra. Growing up in Madagascar, he spoke Malagasy at home, but was forced to speak French at school. He enlisted 25 friends from back home who, over a couple months, contributed enough fine-tuning data to make the model work well in Malagasy. “This is the very first instruction data set for these large language models that includes low-resource languages like Malagasy,” he says. “Malagasy is my mother tongue, so it’s really important for me.”
There are, however, a lot of questions that pop up when guiding the model toward “good” answers. We’re supposed to make sure the grammar of the prompt/completion pairings is correct, but this brings up the thorny question of which dialect of a language we should consider as correct. Some volunteers on the Spanish team are unclear whether they should include separate language categories for Spanish as it is spoken in Spain, Central America, and South America. Other speakers bring up a litany of other questions, familiar to anthropologists but anathema to computational linguists who want unambiguous data: What about the gender of determinants? Or using the formal vous instead of the informal tu? The solution, at least for now, is to conform to the norms of the genre ChatGPT has established, to tap into the voice that is becoming “enregistered” as a recognizable type by quips such as the one by Chris Christie. “What do you expect a model voice to be?” says one manager at Cohere For AI as advice to the confused Spanish-speaking volunteer. “If you’re talking to an algorithm, what would be your expectation of what that tone would be?” he says.
For now, the engineers at Cohere For AI have added a “dialect” tag to the instruction-tuning interface to make the data more navigable, but it’s still a long way from capturing the complexities of language as it is spoken around the world. It might not matter, though. As is often the case in high-stakes science, as it is in political debate, the value lies in how well one does in a trial of strength, not necessarily in how well one agrees with the real world.
Sarah Muir and Michael Wroblewski are the section editors for the Society for Linguistic Anthropology.